ScrAIber
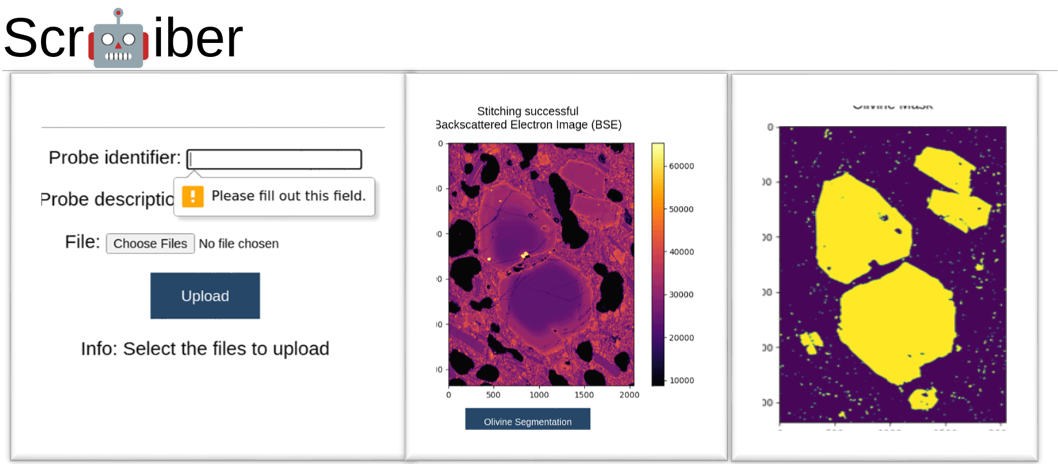
Abstract
An example implementation of automated characterization and interpretation of the textural and compositional characteristics of solids phases in electron microscopy data using machine learning (ML) is presented (http://scraiber.icaml.ikg.uni-hannover.de). In this project we integrated processing pipelines used for scientific work in a convenient web application. This allows to reduce required skills of the users.
In this project, we focus on the characterization of olivine in volcanic rocks, which is a phase that is often chemically zoned. As the olivine crystals represent only less than 10 vol% of the volcanic rock, a pre-processing step is necessary to automatically detect the phases of interest in the images on a pixel level, which is achieved using Deep Learning. A major contribution of the presented approach is to use backscattered electron (BSE) images to: 1.) automatically segment all olivine crystals present in the thin section; and 2.) identify different populations depending on zoning type (e.g., normal vs reversal zoning) and textural characteristics (e.g., microlites vs phenocrysts). The segmentation of the olivine crystals is implemented with a pretrained fully convolutional neural network model with DeepLabV3 architecture. The model is trained to identify olivine crystals in backscattered electron images using automatically generated training data. The training data are generated automatically from images which can easily be created from X-Ray element maps.
Outcome and Trends
The service we provide has been well received by our project partners. This indicates a positive response to our efforts and a strong basis for continued cooperation.
However, we have encountered some challenges. One of the main issues we face is the maintenance of proprietary interfaces. These interfaces, which are essential to our operations, often require specialised knowledge and resources to manage effectively. In addition, the limited generalisation of our current model is another obstacle. Our model, although effective in its current scope, lacks the flexibility to be easily applied to different scenarios or datasets.
In the future, we plan to use the data collected through our service. Our goal is to improve the service by automatically generating reference data. This approach will not only optimise our operations, but also improve the accuracy and reliability of our results. By integrating these data-driven insights into our service, we aim to provide an even more robust and effective solution for our project partners.
Resources
- Live instance
- Code repository (GitLab | Zenodo)
- Final report
- Tutorial
- Container